Cogeneration Power Plants: Improving Operations in Complex Conditions
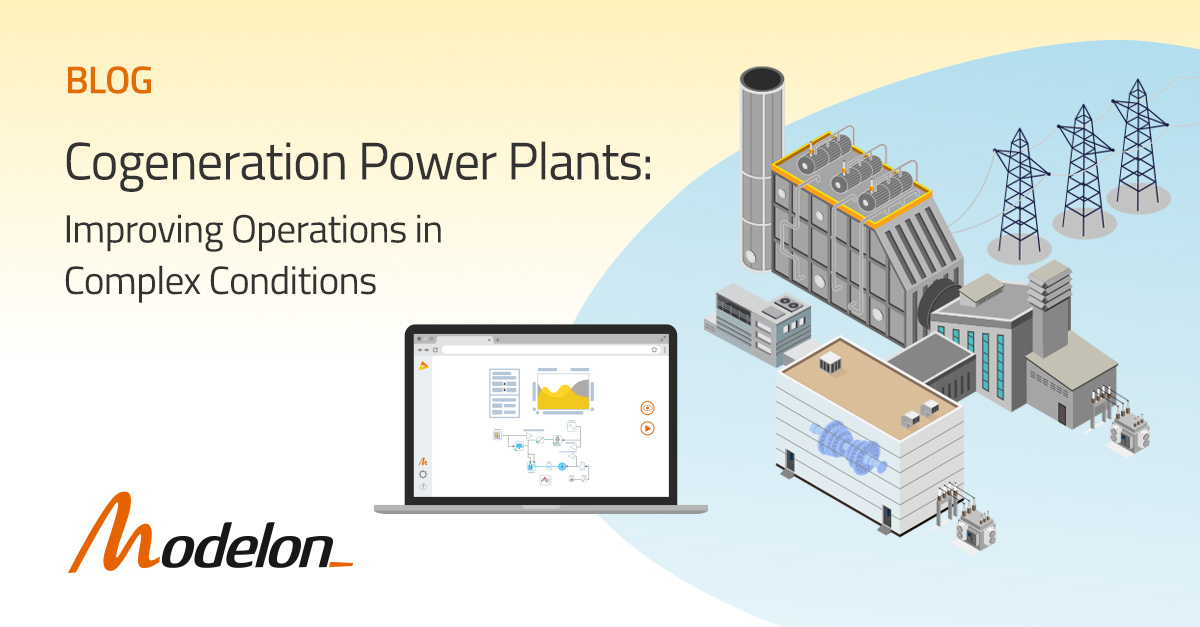
Much of the world’s natural gas power generation infrastructure uses gas turbines connected to Heat Recovery Steam Generators (HRSGs). These HRSGs capture waste heat from gas turbine exhaust and use the heat to generate steam for cogeneration, drive a steam turbine (in a combined cycle plant), or a combination of both. This process recovers most of the thermal energy driving the gas turbine.
On the one hand, it’s wonderful that this technology is so widespread. When evaluated against comparable arrangements in which heat and power generation are separated, cogeneration systems with HRSGs can yield system efficiencies greater than 90%. As such, it’s no surprise that the technology spread so readily during the last decade, with installations surging 30% between 2009 and 2019.
But this can also create obstacles to necessary innovation. Even the most efficient gas turbine/HRSG combined cycle power plant still burns fossil fuels. As decarbonization becomes increasingly urgent, more utilities want to fuel their gas turbines with higher hydrogen blends. A combined cycle gas turbine can start burning hydrogen blends with only modest modification. A natural gas turbine burning a 30% blend of hydrogen (by volume) will emit 11% less CO2.
While the promise is great, existing combined cycle cogeneration plants are inherently complex. Because cogeneration supplies heat and electricity, these plants experience more volatility (i.e., increased load changes). That strains components (driving up maintenance costs) and demands more complex control systems (hindering the development of new solutions). Because these gas turbines are designed to operate for decades and are closely integrated with other components, these layers of interlocking complexity can create a “technological gridlock” that slows innovation.
Embracing model-based design is helping cogeneration plants and engineers break free of this innovation gridlock.
Hydrogen Challenges in Cogeneration Plants
To adapt combined cycle cogeneration plants to hydrogen, teams need to redesign gas turbines and other components to cope with increased wear and adapt the control systems.
Hydrogen burns hotter than natural gas, with an exhaust gas composition higher in moisture and lower in carbon dioxide. This doesn’t just expose a higher threat of condensation in HRSG components; the steam in that exhaust gas can carry more than twice as much heat as the carbon dioxide it’s replacing. Greater heat and moisture will put more wear and tear on HRSG components.
Additionally, unburned hydrogen will always rise to the top of the HRSG in a combined cycle cogeneration facility. If that HRSG was not initially designed for a hydrogen-fed system, it may allow for pockets of hydrogen to collect. As such, the cogeneration plant’s control systems must handle the volatility inherent in cogeneration and add periodic purge cycles for the HRSG to reduce risk.
Developing Controllers for Combined Heat Cogeneration Facilities
The Thermal Power Library (TPL) in Modelon Impact provides a comprehensive modeling, simulation, and optimization framework for exploring cogeneration and combined cycle plant design and operation. It includes ready-to-use models for district heating systems and thermal cogeneration power plants, as well as a complete library of components: boilers, gas turbines, steam turbines, superheaters, evaporators, economizers, duct burners, selective catalytic reduction (SCR) units, thermal energy storage, and more.
By combining existing components in the TPL, engineers and power plant operators can create a multi-fidelity virtual model.
Machine learning (ML) and AI-driven control strategies are a perfect example. ML agents have the potential to react faster than traditional controllers, which can prove vital in these highly dynamic cogeneration systems. With ML, engineers can invest their time and effort in defining how the system should ideally behave rather than getting bogged down with simple heuristics to implement essential performance.
Modelon has partnered with minds.ai to integrate DeepSim (the minds.ai machine learning platform) with Modelon Impact’s models. This has already allowed engineers and researchers to put machine learning to work and test new control strategies on their power plant’s digital twin. For example, in 2021, Ishaan Sood and Jeroen Bédorf used Modelon Impact and minds.ai’s reinforcement learning (RL) agents to generate new, more robust controllers. The AI-guided controller could hew closer to desired setpoints while reacting faster to transient deviations in steam and water flow.
To learn more about Modelon Impact, visit our product page or email us at sales@modelon.com.